Prediction of soil fertility using machine learning in Alto Amazonas province, Peru
DOI:
https://doi.org/10.56926/repia.v3i2.63Keywords:
Random Forest, amazon soils, soil modelling, acid soilsAbstract
The objective of the work was to predict soil fertility in the province of Alto Amazonas with the use of satellite images and machine learning techniques. The study was in the province of Alto Amazonas in Peru. Soil sampling was carried out in all the provinces, totalling 100 samples. Afterwards, soil physical (texture) and chemical analyses were performed. Satellite images were obtained from USGS, and vegetation indexes were calculated based on these images. Finally, descriptive analysis and machine learning modelling using 06 algorithms (GLM, CUBIST, KKNN, SVM, Random Forest and NN) were used and selected based on their R2 and rmse. In this work, we observed that most soils in the province have low pH, P, Mg, K and high acidity. We also managed to achieve good predictions for pH, Ca, Mg and CEC, and we observed that the most successful algorithm was Random Forest. Nevertheless, for Al, CUBIST performed better. This is one of the first works using machine learning to predict soil fertility in the Peruvian Amazon, and we hope it may serve as a base for future projects.
Downloads
References
Arévalo-Gardini, E., Canto, M., Alegre, J., Loli, O., Julca, A., & Baligar, V. (2015). Changes in soil physical and chemical properties in long term improved natural and traditional agroforestry management systems of cacao genotypes in Peruvian Amazon. PLoS ONE, 10(7). https://doi.org/10.1371/journal.pone.0132147 DOI: https://doi.org/10.1371/journal.pone.0132147
Bendig, J., Yu, K., Aasen, H., Bolten, A., Bennertz, S., Broscheit, J., Gnyp, M. L., & Bareth, G. (2015). Combining UAV-based plant height from crop surface models, visible, and near infrared vegetation indices for biomass monitoring in barley. International Journal of Applied Earth Observation and Geoinformation, 39, 79–87. https://doi.org/10.1016/j.jag.2015.02.012 DOI: https://doi.org/10.1016/j.jag.2015.02.012
Brus, D. J. (2019). Sampling for digital soil mapping: A tutorial supported by R scripts. Geoderma, 338, 464–480. https://doi.org/10.1016/J.GEODERMA.2018.07.036 DOI: https://doi.org/10.1016/j.geoderma.2018.07.036
Bünemann, E. K., Bongiorno, G., Bai, Z., Creamer, R. E., De Deyn, G., de Goede, R., Fleskens, L., Geissen, V., Kuyper, T. W., Mäder, P., Pulleman, M., Sukkel, W., van Groenigen, J. W., & Brussaard, L. (2018). Soil quality – A critical review. In Soil Biology and Biochemistry (Vol. 120, pp. 105–125). https://doi.org/10.1016/j.soilbio.2018.01.030 DOI: https://doi.org/10.1016/j.soilbio.2018.01.030
Canero, F. M., Rodriguez-Galiano, V., & Aragones, D. (2024). Machine Learning and Feature Selection for soil spectroscopy. An evaluation of Random Forest wrappers to predict soil organic matter, clay, and carbonates. Heliyon, 10(9). https://doi.org/10.1016/j.heliyon.2024.e30228 DOI: https://doi.org/10.1016/j.heliyon.2024.e30228
Campos, A. R., Giasson, E., Costa, J. J. F., Machado, I. R., Silva, E. B. da, & Bonfatti, B. R. (2019). Selection of Environmental Covariates for Classifier Training Applied in Digital Soil Mapping. Revista Brasileira de Ciência Do Solo, 42, e0170414. https://doi.org/10.1590/18069657RBCS20170414 DOI: https://doi.org/10.1590/18069657rbcs20170414
Chen, J. M. (1996). Evaluation of vegetation indices and a modified simple ratio for boreal applications. Canadian Journal of Remote Sensing, 22(3), 229–242. https://doi.org/10.1080/07038992.1996.10855178 DOI: https://doi.org/10.1080/07038992.1996.10855178
Chuvieco, E., Martín, M. P., & Palacios, A. (2002). Assessment of different spectral indices in the red-near-infrared spectral domain for burned land discrimination. International Journal of Remote Sensing, 23(23), 5103–5110. https://doi.org/10.1080/01431160210153129 DOI: https://doi.org/10.1080/01431160210153129
Dash, J., & Curran, P. J. (2007). Evaluation of the MERIS terrestrial chlorophyll index (MTCI). Advances in Space Research, 39(1), 100–104. https://doi.org/10.1016/j.asr.2006.02.034 DOI: https://doi.org/10.1016/j.asr.2006.02.034
Delgado-Caballero, C. E., Gómez-Guerrero, A., Valdez-Lazalde, J. R., De los Santos- Posadas, H., Fierros-González, A. M., & Horwath, W. R. (2009). Site index and soil properties in young plantations of Eucalyptus grandis and E. urophylla in southeastern México. Agrociencia, 43(1). https://www.agrociencia-colpos.org/index.php/agrociencia/article/view/697
Dharumarajan, S., Lalitha, M., Niranjana, K., & Hegde, R. (2022). Evaluation of digital soil mapping approach for predicting soil fertility parameters—a case study from Karnataka Plateau, India. Arabian Journal of Geosciences, 15(5), 1–21. https://doi.org/10.1007/S12517-022-09629-8 DOI: https://doi.org/10.1007/s12517-022-09629-8
Di Raimo, L. A. D. L., Couto, E. G., de Mello, D. C., Demattê, J. A. M., Amorim, R. S. S., Torres, G. N., Bocuti, E. D., Veloso, G. V., Poppiel, R. R., Francelino, M. R., & Fernandes-Filho, E. I. (2022). Characterizing and Modeling Tropical Sandy Soils through VisNIR-SWIR, MIR Spectroscopy, and X-ray Fluorescence. Remote Sensing 2022,14(19), 4823. https://doi.org/10.3390/RS14194823 DOI: https://doi.org/10.3390/rs14194823
EMBRAPA. (2009). Manual de análises químicas de solos, plantas e fertilizantes (2nd ed.). Embrapa. www.sct.embrapa.br/liv
Frampton, W. J., Dash, J., Watmough, G., & Milton, E. J. (2013). Evaluating the capabilities of Sentinel-2 for quantitative estimation of biophysical variables in vegetation. ISPRS Journal of Photogrammetry and Remote Sensing, 82, 83–92. https://doi.org/10.1016/j.isprsjprs.2013.04.007 DOI: https://doi.org/10.1016/j.isprsjprs.2013.04.007
Gamon, J. A., & Surfus, J. S. (1999). Assessing leaf pigment content and activity with a reflectometer. New Phytologist, 143(1), 105–117. https://doi.org/10.1046/j.1469-8137.1999.00424.x DOI: https://doi.org/10.1046/j.1469-8137.1999.00424.x
Gitelson, A. A., Gritz, Y., & Merzlyak, M. N. (2003). Relationships between leaf chlorophyll content and spectral reflectance and algorithms for non-destructive chlorophyll assessment in higher plant leaves. Journal of Plant Physiology, 160(3), 271–282. https://doi.org/10.1078/0176-1617-00887 DOI: https://doi.org/10.1078/0176-1617-00887
Gitelson, A. A., Merzlyak, M. N., & Chivkunova, O. B. (2001). Optical Properties and Nondestructive Estimation of Anthocyanin Content in Plant Leaves. Photochemistry and Photobiology, 74(1), 38–45. https://doi.org/10.1562/0031-8655(2001)0740038opaneo2.0.co2 DOI: https://doi.org/10.1562/0031-8655(2001)074<0038:OPANEO>2.0.CO;2
Hounkpatin, K. O. L., Bossa, A. Y., Yira, Y., Igue, M. A., & Sinsin, B. A. (2022). Assessment of the soil fertility status in Benin (West Africa) – Digital soil mapping using machine learning. Geoderma Regional, 28, e00444. https://doi.org/10.1016/J.GEODRS.2021.E00444 DOI: https://doi.org/10.1016/j.geodrs.2021.e00444
Huete, A. R. (1988). A soil-adjusted vegetation index (SAVI). Remote Sensing of Environment, 25(3), 295–309. https://doi.org/10.1016/0034-4257(88)90106-X INEI. (2012). IV Censo Nacional Agropecuario . In Resultados Definitivos. IV Censo Nacional Agropecuario. http://proyectos.inei.gob.pe/web/documentospublicos/resultadosfinalesivcenagro.pdf DOI: https://doi.org/10.1016/0034-4257(88)90106-X
Jordan, C. F. (1969). Derivation of Leaf-Area Index from Quality of Light on the Forest Floor. Ecology, 50(4), 663–666. https://doi.org/10.2307/1936256 DOI: https://doi.org/10.2307/1936256
Köppen, W. (1931). Grundriß der Klimakunde. In Grundriß der Klimakunde. De Gruyter. https://doi.org/10.1515/9783111667751 DOI: https://doi.org/10.1515/9783111667751
Lang, P., Qin, C. zhi, Zhu, A. xing, Hou, Z. wei, Fan, N. qing, & Wang, Y. jie. (2020). A case- based method of selecting covariates for digital soil mapping. Journal of Integrative Agriculture, 19(8), 2127–2136. https://doi.org/10.1016/S2095-3119(19)62857-1 DOI: https://doi.org/10.1016/S2095-3119(19)62857-1
Louhaichi, M., Borman, M. M., & Johnson, D. E. (2001). Spatially located platform and aerial photography for documentation of grazing impacts on wheat. Geocarto International, 16(1), 65–70. https://doi.org/10.1080/10106040108542184 DOI: https://doi.org/10.1080/10106040108542184
Lu, Q., Tian, S., & Wei, L. (2023). Digital mapping of soil pH and carbonates at the European scale using environmental variables and machine learning. Science of The Total Environment, 856, 159171. https://doi.org/10.1016/J.SCITOTENV.2022.159171 DOI: https://doi.org/10.1016/j.scitotenv.2022.159171
Lymburner, L., Beggs, P. J., & Jacobson, C. R. (2000). Estimation of canopy-average surface-specific leaf area using Landsat TM data. In Photogrammetric Engineering and Remote Sensing (Vol. 66, Issue 2, pp. 183–191).
Macedo Neto, A. A. L.; Farias, P. R. S.; Matos, G. S. B.; Silva, G. B.; Santos, A. V. F. Anhê, B. B. (2020). Diagnosis and spatial variability of soil fertility and crop production in a teak area in Eastern Pará State. CERNE, 26(1), 37-47. https://doi.org/10.1590/01047760202026012683 DOI: https://doi.org/10.1590/01047760202026012683
Mosaid, H., Barakat, A., John, K., Faouzi, E.H., Bustillo, V., El Garnaoui, M., & Heung, B. (2024). Improved soil carbon stock spatial prediction in a Mediterranean soil erosion site through robust machine learning techniques. Environmental monitoring and assessment, 196 2, 130. https://doi.org/10.1007/s10661-024-12294-x DOI: https://doi.org/10.1007/s10661-024-12294-x
Qi, J., Chehbouni, A., Huete, A. R., Kerr, Y. H., & Sorooshian, S. (1994). A modified soil adjusted vegetation index. Remote Sensing of Environment, 48(2), 119–126. https://doi.org/10.1016/0034-4257(94)90134-1 DOI: https://doi.org/10.1016/0034-4257(94)90134-1
R Core Team. (2021). R Core Team (2021). R: A language and environment for statistical computing. R Foundation for Statistical Computing, Vienna, Austria. URL Http://Www.R-Project.Org/
Richardson, A. J., & Wiegand, C. L. (1977). Distinguishing Vegetation from Soil Background Information. A gray mapping technique allows delineation of any Landsat scene into vegetative cover stages, degrees of soil brightness, and water. Photogrammetric Engineering and Remote Sensing, 43(13), 1541-1552. https://www.asprs.org/wp- content/uploads/pers/1977journal/dec/1977_dec_1541-1552.pdf
Rondeaux, G., Steven, M., & Baret, F. (1996). Optimization of soil-adjusted vegetation indices. Remote Sensing of Environment, 55(2), 95–107. https://doi.org/10.1016/0034-4257(95)00186-7 DOI: https://doi.org/10.1016/0034-4257(95)00186-7
Rouse, J. W., Haas, R. H., Schell, J. A., & Deering, D. W. (1974). Monitoring vegetation systems in the great plains with ERTS. NASA Goddard Space Flight Center 3d ERTS-1 Symposium, 1, 309–317. https://doi.org/10.1021/jf60203a024 DOI: https://doi.org/10.1021/jf60203a024
Roy, D. P., Boschetti, L., & Trigg, S. N. (2006). Remote sensing of fire severity: Assessing the performance of the normalized burn ratio. IEEE Geoscience and Remote Sensing Letters, 3(1), 112–116. https://doi.org/10.1109/LGRS.2005.858485 DOI: https://doi.org/10.1109/LGRS.2005.858485
Siqueira, R. G., Moquedace, C. M., Francelino, M. R., Schaefer, C. E. G. R., & Fernandes- Filho, E. I. (2023). Machine learning applied for Antarctic soil mapping: Spatial prediction of soil texture for Maritime Antarctica and Northern Antarctic Peninsula. Geoderma, 432, 116405. https://doi.org/10.1016/J.GEODERMA.2023.116405 DOI: https://doi.org/10.1016/j.geoderma.2023.116405
Sripada, R. P., Heiniger, R. W., White, J. G., & Meijer, A. D. (2006). Aerial color infrared photography for determining early in-season nitrogen requirements in corn. Agronomy Journal, 98(4), 968–977. https://doi.org/10.2134/agronj2005.0200 DOI: https://doi.org/10.2134/agronj2005.0200
Sujatha, M., & Jaidhar, C. D. (2023). Machine learning-based approaches to enhance the soil fertility—A review. Expert Systems with Applications, 240, 122557. https://doi.org/10.1016/j.eswa.2023.122557 DOI: https://doi.org/10.1016/j.eswa.2023.122557
Tucker, C. J. (1980). A spectral method for determining the percentage of green herbage material in clipped samples. Remote Sensing of Environment, 9(2), 175–181. https://doi.org/10.1016/0034-4257(80)90007-3 DOI: https://doi.org/10.1016/0034-4257(80)90007-3
Vescovo, L., & Gianelle, D. (2008). Using the MIR bands in vegetation indices for the estimation of grassland biophysical parameters from satellite remote sensing in the Alps region of Trentino (Italy). Advances in Space Research, 41(11), 1764–1772. https://doi.org/10.1016/j.asr.2007.07.043 DOI: https://doi.org/10.1016/j.asr.2007.07.043
Vieira, R. M. da S. P., Tomasella, J., Barbosa, A. A., Polizel, S. P., Ometto, J. P. H. B., Santos, F. C., Ferreira, Y. da C., & Toledo, P. M. de. (2021). Land degradation mapping in the MATOPIBA region (Brazil) using remote sensing data and decision-tree analysis. Science of The Total Environment, 782, 146900. https://doi.org/10.1016/J.SCITOTENV.2021.146900 DOI: https://doi.org/10.1016/j.scitotenv.2021.146900
Wadoux, A. M. J. C., Minasny, B., & McBratney, A. B. (2020). Machine learning for digital soil mapping: Applications, challenges and suggested solutions. Earth-Science Reviews, 210, 103359. https://doi.org/10.1016/J.EARSCIREV.2020.103359 DOI: https://doi.org/10.1016/j.earscirev.2020.103359
Wang, F., Huang, J., Tang, Y., & Wang, X. (2007). New Vegetation Index and Its Application in Estimating Leaf Area Index of Rice. Rice Science, 14(3), 195–203. https://doi.org/10.1016/s1672-6308(07)60027-4 DOI: https://doi.org/10.1016/S1672-6308(07)60027-4
Watt, M. S., Pearse, G. D., Dash, J. P., Melia, N., & Leonardo, E. M. C. (2019). Application of remote sensing technologies to identify impacts of nutritional deficiencies on forests. ISPRS Journal of Photogrammetry and Remote Sensing, 149, 226–241. https://doi.org/10.1016/j.isprsjprs.2019.01.009 DOI: https://doi.org/10.1016/j.isprsjprs.2019.01.009
Wu, W. (2014). The Generalized Difference Vegetation Index (GDVI) for dryland characterization. Remote Sensing, 6(2), 1211–1233. https://doi.org/10.3390/rs6021211 DOI: https://doi.org/10.3390/rs6021211
Yang, L., Li, X., Shi, J., Shen, F., Qi, F., Gao, B., & Chen, Z. (2020). Geoderma Evaluation of conditioned Latin hypercube sampling for soil mapping based on a machine learning method. Geoderma, 369, 114337. https://doi.org/10.1016/j.geoderma.2020.114337 DOI: https://doi.org/10.1016/j.geoderma.2020.114337
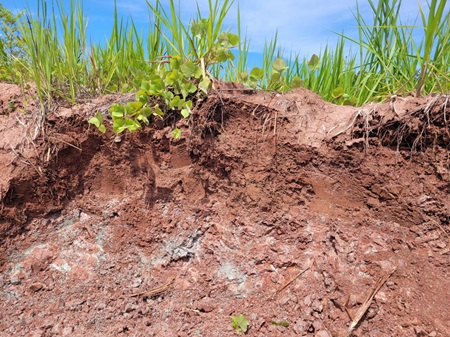
Published
How to Cite
Issue
Section
License
Copyright (c) 2024 César Oswaldo Arévalo-Hernández, Enrique Arévalo-Gardini, Luis Alberto Arévalo-López, Oscar Tuesta-Hidalgo, Dayani Shirley Romero-Vela, Claudia Elizabeth Ruiz-Camus

This work is licensed under a Creative Commons Attribution 4.0 International License.
Authors retain their rights:
a. The authors retain the intellectual property rights (copyright) of the published works, assigning to the journal the right of first publication.
b. Authors retain their trademark and patent rights, and also on any process or procedure described in the article.
c. Authors retain the right to share, copy, distribute, perform and publicly communicate the article published in REPIA (e.g., place it in an institutional repository or publish it in a book), with an acknowledgement of its initial publication in REPIA.
d. Authors retain the right to make a subsequent publication of their work, to use the article or any part of it (e.g., a compilation of their work, notes for conferences, theses, or for a book), provided they indicate the source of publication (authors of the work, journal, volume, number, and date).